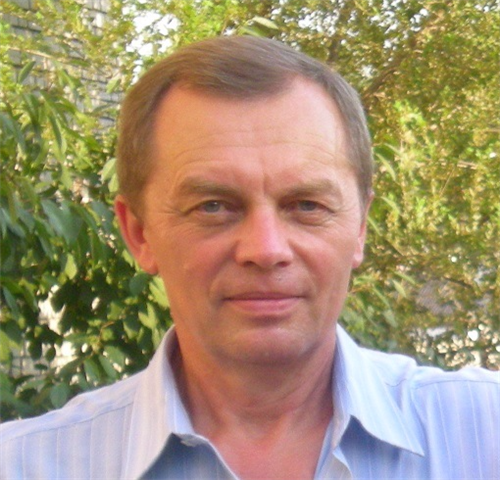
shegenn 10.06.2020
“Statistical Thinking” and “Big Data”
“Big Data” is an important new trend related to the collection and analysis of huge data sets, for example, such as those that cannot be contained by an ordinary computer. It opens up the incredible possibilities to almost all areas of human activity.
The supporters of this trend believe with good reason that these openings will radically change our lives. However, in the euphoria of the openings, it seems to many of us that all old theoretical models related to the data collection and analysis can now be dismissed, and it would be sufficient to use a simple correlation analysis. Meanwhile, the supporters of the statistical thinking principles have another vision. They believe anyway that some discipline will be necessary maintained in the approach to data bulk collecting and analyzing. This work is aimed at discussing this divisive issue. We believe that the “statistical thinking” point of view should not be neglected.
|
“All models are wrong, but some are useful.” G. Box “The purpose of calculations – not numbers, but understanding.” R. Hamming “The combination of phenomena causes is not available for the human mind. But the need to find reasons is put into the person’s soul.” L. Tolstoy |
With the growth of technical capabilities, there is an increase demand in their using among people. It is easy to see in the Big Data example. As soon as data collection fell in price sharply due to numerous sensors, barcodes and embedded computers, the idea of such software products that make it possible to combine a large number of ordinary computers into a single network came up; and such a network is capable to process huge amount of data; this is inconceivably for ordinary computers. Moreover, it was possible to lower the requirements sharply for homogeneity and sequences of the collected data, and, therefore, significantly cut the expenses. And it is about more than the costs, as new opportunities that really change almost everything that surrounds us are opening up.
It seems to the new approach adherents that many classical methods of data collection and analysis can be forgotten, along with many problems of data collection and analysis that have accumulated over many decades. See, for example, Chris Anderson, editor-in-chief of Wired Magazine, says in summer 2008 (we quote the title of his work) [1]: “The End of Theory: The Data Deluge Makes the Scientific Method Obsolete.” Chris wrote then: “Sixty years ago, digital computers made information readable. Twenty years ago, the Internet made it reachable. Ten years ago, the first search engine crawlers made it a single database. Now Google and like-minded companies are sifting through the most measured age in history, treating this massive corpus as a laboratory of the human condition. We are the children of the Petabyte Age.” Many things are different than before in this era. Kilobytes were stored on floppy disks; a hard disk was needed to store megabytes, a secondary storage device or a drive array subsystem with two or more disk drives were already needed to store terabytes; and petabytes are stored in the “clouds”. Special cloud storages had to be developed in 2005-2010 in order to store arrays of this size (see, for example, [2]).
Peter Norvig, Google's research director, when opening one of the conferences, citing our first epigraph, said: “All models are wrong, and increasingly you can succeed without them.” Listing such scientific achievements as Newton’s laws of motion, quantum physics, Darwin’s theory, genetics, Chris [1] says that only particular models are created in all these sciences; such models are constantly revised and become so complicated with time that working with them becomes more and more expensive, and the answers are less and less interesting. In his opinion, the human and society sciences did not move forward to their objects understanding at all. “Who knows why people do what they do?” he asks. His article ends with a rhetorical question: “What can science learn from Google?” We will get back to this grand question. In the meantime, let us find out: what kind of magical weapons does Google have that serves it as “open sesame!” that opens any door? It appears that this information is not new; this is the most common correlation but it is applied to large data sets. “Correlation is enough” [1]. Although Chris softened his stance later, the key questions remained. And we will discuss them after referring to the book [3] that is the first book about Big Data translated into Russian. The authors are on the same side of the fence as Chris, but they argue their viewpoint in more detail as far as this is a book. Here is a brief account of the Correlation chapter highlights.
There is a main question, and scientific research was carried out in the past in order to answer it. This question is “Why?”, and it requires the assumption of the causal relationship existence between the phenomena studied. If we believe in causality, then it is natural to implement a process: hypothesizing – testing of a hypothesis at every possible way – new hypothesis formation, as the previous one is rarely reasonable. It is long, expensive and not effective. The main difficulty is that the initial question is not posed correctly. Instead of “Why?” it should be asked “What?”. Then we immediately refuse to be on the hunt for causality; and instead of this exercise in futility, we conduct pioneer research in the correlations between many millions of variables in arrays not only of large dimension, but also with a huge number of implementations (observations, experiments, events). And then, as if by magic, the answers that we need appear. The obtained correlation set allows making forecasts with a high probability of success, and this is the success formula in using Big Data. Of course, nonlinear correlations are sometimes useful, but this is a matter for the future. The authors of [3] further discuss the problem of causality with the reference to the work of American psychologist and economist Daniel Kahneman who the Nobel Prize laureate is. According to them, there are two ways of the world understanding:
- through the quick illusory causal relationships, and
- through a slow methodological casual experiment.
According to D. Kahneman, this corresponds to two forms of reasoning that are fast and slow. Moreover, the authors of [3] supposed the inner sense of causality does not deepen our world understanding. This is just an illusion of understanding.
Let us get down to our second epigraph now. Practically nothing follows from the fact that we measured or calculated something. The calculation result must be introduced into a certain context, only then it can be used for some reason. For example, if we determined that the height of a certain person is two meters and fifty centimeters, this is only a statement in itself. However, if we find out that people higher than two meters are uncommon, and there is no such a human height these days, then we understand that either a mistake creeped into the result or a unique person was found. This is called understanding in ordinary language. It is far from the causality problems and associated philosophical reasoning. To understand means to put in context and have the opportunity to make decisions. Moreover, this context may be inadequate, and decisions may prove to be erroneous. But people do not know how to act otherwise. They will perceive the discovered errors as a source of information, which should facilitate the transition to a new, deeper level of understanding. This is called life experience in everyday life.
It happened that at first, correlation analysis was useful to solve the problems associated with Big Data. This is one of the most common tools for applied statistics. Apparently, Georges Cuvier introduced it into European science in 1806. He was interested, for example, in the animal skeleton possible reconstruction by separate elements in archeology and paleontology. Nevertheless, he had many predecessors. It is useful enough to recall Gulliver, whom only the right thumb diameter at the bottom was practically measured to in order to sew a shirt, as Cuvier-style correlation was already known in Laputa in 1720 (when Jonathan Swift’s novel was published). It was put into statistical practice by Francis Galton in 1888, and carefully described mathematically in the last years of the 19th century by Karl Pearson. A lot of water has passed under the bridge since that time. A correlation assessment method dependence on the scale types was found where the task variables are described. Many correlation coefficients and statistical criteria for testing of hypotheses significance are considered in [4]. Experience with correlations has shown that the key problem is “false” correlations. This problem is perfectly illustrated by the next statistical thin story. When the statisticians got to the resident registers of Stockholm, they found a wide variety of records there over the past hundred years. They were interested in data on the number of newborns in the families of this city, and on the number of storks that were registered according to the rules. The correlation between these two indicators was almost undistinguishable from unity! Finally, it was possible to “prove” scientifically that storks bring children. Supporters to the view that children are found under a gooseberry bush were put to shame. This is the power of correlation. It is clear that the secret is simple in this case: both children and storks depend on the material well-being level of residents.
We are interested in multidimensional correlations in the context of Big Data. The statistics also dealt with them. At the beginning of the 20th century, British psychologists studied whether student performance in one subject depends on success in other subjects. It all started with C. Spearman’s works in 1906. Gradually, it has faded to a huge field of applied multidimensional statistics that is called factor analysis [5]. The factor analysis is characterized by the ambiguity of the results and their interpretation difficulty. Eventually, Big Data seems to be faced with similar problems. A book on correlation for non-statisticians has recently appeared [6]. The moral is simple: correlation is a complex and tricky tool that produces mixed results. It is tempting to interpret it as causal, although it is clear that it is dangerous. What is known as correlations in Big Data and calculated by the correlation coefficient formula is essentially a certain measure that is proportional to the angle cosine between multidimensional vectors, that is, a measure of the vector collinearity. It becomes a measure of correlation only within a certain statistical model framework. Thus, we get vector sheaves that are at small angles with each other and, accordingly, close to orthogonal ones to them. And, of course, all the intermediate options. It is entirely possible that this kind of information is useful.
Now it is necessary to return to determinism and to the opposed models to it. First, let us address to our third epigraph. As usual, Leo Tolstoy generalizes the situation with causality successfully. Of course, the interminable controversy between Laplacian determinism (“give me the coordinates and velocities of all particles in the universe, and I will accurately predict their past, present and future”) and stochastic models of the world (for example, “the world is a network, gems are located in its nodes, and each gem reflects all the others and is reflected in all the others”) will never end. Although, there is convincing evidence that stochastic ideas dominate in the microworld. Niels Bohr wrote this in 1949: “The question at issue has been whether the renunciation of a causal mode of description of atomic processes involved in the endeaverours to cope with the situation should be regarded as a temporary departure from ideals to be ultimately revived or whether we are faced with an irrevocable step towards obtaining the proper harmony between analysis and synthesis of physical phenomena” [7].
Laplace believed that the world is determinate, and individual errors, failures; inaccuracies are simply natural consequences of the world complexity and our inability to absolute knowledge. After the quantum world birth, such a position becomes difficult to defend. Therefore, V. V. Nalimov [8] developed the concept of a probabilistic vision of the world at the end of the last century. That is what he writes (p. 17): “We are talking not only about the probabilistic vision of the World associated with its infinite complexity, but “in fact” internally deterministic, namely the probabilistic World, where the probability is in the essence. This is the probabilistic ontology of the probabilistic World, and not the probabilistic epistemology of the deterministic World.”
Thus, we can assume that the balance tips toward probabilistic representations that contribute to Big Data, primarily due to its size. It seems that Big Data has not changed the situation with causality, as it was not good enough before too. It seems that now it is impossible to interpret the results meaningfully. We think that the plain fact is that the experience to provide explanations has not been gained yet. Apparently, it will be gained soon. As you know, you can explain everything post factum if required. As for the models, it seems that we are also dealing with a misperception. Theoretical models were very rare previously. Indeed, it comes as no surprise that Norbert Wiener proposed a black box model, which expects that we have no theoretical considerations at all. Particularly, such a model is used in Big Data, but without a name. There is no reason to talk about “the end of the theory” just because the black box model is used. After all, there are other scientific reasons. For example, it is enough to make reference to Philipp Frank [9] in order to find out that there are so-called intelligible principles that are not derived from experience, but subordinated to the experience results. For example, they include conservation laws, the second law of thermodynamics, and other concepts. Setting about the Big Data analysis you do not annul the Pythagorean theorem or Snell's law of refraction. Therefore, we are sure that science will always have something to say to Google.
Now we are ready to discuss the role of statistical thinking. In this case, we will rely on work [10]. In spite of the mainstream thinking, we assume that for Big Data purposes we should get as close to statistical thinking as possible. Indeed, such a missing foundations will arise then. And everyone will win. How can statistical thinking help? Perhaps, this is partly because of the advertising nature of many publications on the Big Data analysis, but it is still not clear as to the methodology for information collecting and analysis. In this situation, statistical thinking with its discipline should help a systematic approach. How many variables are worth considering? Which ones? What scales do they have to be represented in? The idea that all this can be done “anyhow” is beneath criticism. One of the key concepts of statistical reasoning is variability. It is inherent in all processes, both natural (then it is often called changebility) and created by people. Incidentally, no matter how long the observation of a process that changes only within its natural variation, that is scarcely change, does not create significant information about this object. All that can be learned from such an observation is its average value estimation (for Big Data, estimation of mean is very reliable, or, as they say, a measure of the central tendency) and some variability measure estimation (for example, the squared error). Notably, it is probably that some significant correlations will come to light; however, they will likely be false, unfortunately. There it is the direct benefit from statistical thinking.
Another important concept of statistical thinking is a data generating process. It is conceivable the data generated by several different processes. In all cases, it is important for us to find out: How do these processes function? Are they in statistically controlled states? Shewhart charts or other similar tools are usually used to answer such questions. If the statistical controllability hypothesis of the studied process (object) is not rejected as a result of long-term observation, then the additional artificial variation is worth introducing into the corresponding processes in order to obtain information about the subject of our interest. This means that we must fall back on the experiment planning methods. And we think that is exactly the way to act. Concerning the quantity and types of variables (factors) that should be considered, it is important to remember that we are usually unsuspicious of what factors should be considered. I dealt with a large manufacturer of artificial fiber many years ago. One of its important parameters (characteristics) for consumers was high strength of fibers. The fiber forming process was carried out at temperatures exceeding 1500°C, but strength of fibers strongly correlated with the climatic conditions of the area where the plant was located, primarily with temperature and humidity as we accidentally discovered. It was difficult to explain, and we did not succeed in it, but the regularities were observed with high accuracy for several years (in the past). Besides, we had enough data from the workshop laboratory and the local meteorological center. So, the question of factors selection remains open, despite the simplification and cheapening of data acquisition procedures. Note that one specific problem requires discussion. The identification and evaluation of the response or responses occurs by itself in some tasks. But there are also other tasks when goal functions are not required. Such a difference can lead to far-reaching consequences.
Further, metrology starts to set in. What scales are the factors measured in? What devices? What are measurement errors? Is there a stable measuring system? Correlations are especially sensitive to the choice of scales. The same factor can be measured in hundreds of scale models. Correlation is not indifferent to the choice of scale model. It is not a problem for Big Data to take into account the “human factor” function during the measurement process. For example, there is no doubt that the operator conducting the chemical analysis contributes to the accuracy of the result and other metrological characteristics. Up to the present time, it was difficult to take into account factors of this kind, and not for “ideology” reasons, but for purely technical reasons. Now, it is hoped that such problems will disappear.
The authors of [10] suggest this sequence of actions when working with Big Data.
- Accurate statement of the problem.
- Process understanding.
- Analysis strategy development.
- Search for sources of variation.
- Data quality assessment.
- Deep subject knowledge.
- Step-by-step approach.
- Process modeling.
The term “statistical thinking” may be misleading itself. As noted in [10], it refers to the way we think about problems and apply statistics to them, but not to algorithms, equations, and even data. This is not a methodology, but a philosophy. Statistics Department of American Society for Quality formulated the standard definition of this term in the mid-90s that resolves itself into this:
- All works take place in a system of interconnected processes.
- Variation is inherent in all processes.
- Understanding and decrease in variation is the key to success.
Following on from [10], let us consider the problem of data quality evaluation as an example. It is clear that, the analysis of data origin is the beginning of every analysis. If there is a great deal of data, it is significantly complicated to study it. Indeed, there is no visual control possibility now; data from different sources are often mixed, so that a mixture of “apples with oranges” is obtained; missing data occur almost inevitably, when it is not clear what to do with them; systematically, the data are subjected to automatic clearing that is very dangerous because it is easy to “throw out the baby along with the water”; and this listing can be continued.
Special consideration of all subjective data related to the experience of specialists in the object field under study, the opinions of experts in related fields in order to solve the problem, as it is clear that Big Data rarely belongs into a narrow knowledge area entirely. So, this is about teamwork on each project. And this again poses a new task of team members training, common terminology developing and continuing dialogue organizing.
Experience shows that the project success of such complexity is achieved through a consistent approach to research. PDCA (Shewhart-Deming cycle) is quite appropriate here.
A more detailed analysis is outside our plans; it was enough for us to show that statistical thinking has something to share with Big Data. Together they can realize more than individually. A review on early works on Big Data was published in [11].
References
- Anderson C. The end of theory: the data deluge makes the scientific method obsolete. // Wired Magazine, June 23, 2008. Available at: www.wired.com/science/dis coveries/magazine/16-07/pb_theory. (Accessed January 11, 2014).
- Cherniak L. Integration Is a Cloud Basis // Otkrytye sistemy [Open Systems], 2011. - № 7. – September 16th. [In Russian]
- Mayer-Schönberger V., Cukier K. Big Data: A Revolution that Will Transform How We Live, Work, and Think. / Translated from English by Ynna Gaidiuk. – Moscow: Mann, Ivanov and Farber, 2014. – 240 p. [In Russian]
- Glass G., Stanley J. Statistical Methods in Education and Psychology. / Translated from English; under general editorship of Y. Adler. Moscow: Progress, 1976. – 495 p. [In Russian]
- Lawley D., Maxwell A. Factor Analysis as a Statistical Method. – Translated from English by N. Blagoveshhenskii. – Moscow: Mir, 1967. 144 p. [In Russian]
- Blagoveshhenskii Y.N. Secrets of Correlation Relationships in Statistics. – Moscow: Nauchnaya kniga: INFRA-M, 2009. – 158 p. [In Russian]
- Bohr N. Discussion with Einstein on epistemological problems in atomic physics –In: Atomic physics and human cognition. – Moscow: Nauka, 1961. – 151 p. [In Russian]
- Nalymov V. V., Dragalyna Zh. A. The Reality of the Unreal. A Probabilistic Model of the Unconscious. – Moscow: Izdatelstvo «Mir Idej», AO AKRON, 1995. – 432 p. [In Russian]
- Frank P. Philosophy of Science: The Link Between Science and Philosophy./ Translated from English; under general editorship of A. Kursanov. – Moscow: Izdatelstvo LKI, 2010. – 512 p. [In Russian]
- Roger W. Hoerl, Ronald D. Snee and Richard D. De Veaux //Applying statistical thinking to ‘Big Data’ problems. WIREs Computational Statistics. -Volume 6. - July/August 2014. – P. 222-232.
- Adler Y. P., Chernykh E. A. Statistical process control. Big Data. – Moscow: MISIS, 2016. -52 p. [In Russian]