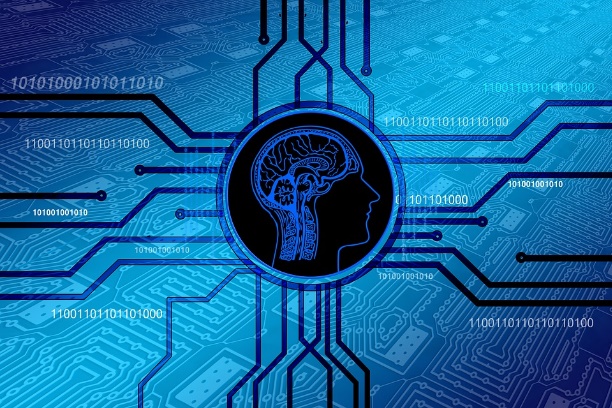
ScienceHunter 21.06.2019
Methods of data science in social research
What risks are linked to the application of data science in the study of society?
- What are the main risks associated with the popularity of Big Data methods in social research?
- The book “Bit by Bit” by Matthew Salganik was released in 2017. It is dedicated to the possibilities of computational methods using in the social sciences. Salganik differs two types of research approaches: question-driven. The research related to data science is data-driven. Many people confirm that the study of digital traces in the social space is the mainstream of social science in the near future.
The first risk arises from the word “mainstream” as we are in a situation of reduced methodological diversity. A certain way of research is approved almost as a normative one. But the methods of data science allow you to make predictions but they often make it impossible to build the explanation and the interpretation. Thus, the question of a method choosing is concerned with the choice of a specific idea about the scientific knowledge functions.
The second problem of turning to computational social science is that data scientists may be so far from the data taken context. The beauty of the model replaces the contextual connection and the clear relationship between the organization of the theoretical object and the reality that this object represents. The fact that the representation of a certain "reality" is required from the data science is due to the fact that this is the way the funding institutions and decision makers that are policymakers themselves see its usefulness.
The third problem is particularly visible in the corporate sector: the data can’t answer all the questions. They often do not respond in the way that we would like to. Data researchers don’t have the opportunity to defend in a well-argued manner against political pressure, the management pressure which want to insert the interpretation of this data into a certain system of decisions at the same time. In this sense, the turn to data makes users behavior is more transparent but does not protect us from its deformed reading in order to serve a specifically understood spectrum of goals.
- What does your statement that data science is incapable of giving theoretical explanations mean?
- I would put this statement more carefully. Data science has a rich explanatory potential. However, while we often hear the statement that at first you need to collect as much data as it is possible and on its basis you can theorize. Chris Anderson, the editor of Wired put forward such ideas in 2008 in his article “The End of Theory”. Franco Moretti says in his book “Distant Reading” which was translated into Russian: it is not the time for theory now; perhaps you should not be overly committed to the ideal of a theoretically loaded and critically thinking science. Although it must be admitted that left-wing intellectuals wanted to achieve the liberation from theory in the 20th century.
The problem is that the statement about the postponement of theoretical reflection has consequences outside the academic community. The researchers sooner or later go to the level of this knowledge consumers represented by states, corporations, the general public and when they hear the thesis about the uselessness of the theory, they often interpret it as the long-known truth confirmation that academicians are useless "in practice". Their benefit is only in collecting data, but they can’t tell us anything about what should be done. In this sense, the problem of the theory absence is how this fact is accepted broadly. It can be read as a scientists remission of the claims to for the influence in a public context.
Neither Moretti, nor theorists of Digital Humanities, nor, Paul Dorish, who published the book “What the Social Action is” in 2004, pretend to supply the theory with an integrated critical module. This theory can work on mathematical and algorithmic models, but it differs a lot from many social and humanitarian theories of the past as it is literally uncritical. There was the journal issue Critical Inquiry, it seems, in 2007, where Bruno Latour said: "Criticism is no longer needed, this is an exhausted procedure." Although he is not a representative of Data Science or Computational Social Science but this statement coincides with the general ideology of this movement.
- The researchers remoteness from the context suggests associations with colonial knowledge that is devoid of local context implementing the same rules and norms. What extent do Data Science research practices inherit this principle to?
- It is interesting to read in colonial terms. Thanks to Andre Gortsu and others, we know that modern capitalism is immaterial. In this sense, data capitalism leads to the immaterial colonialism formation where not only the territories are colonized but also the knowledge systems, the channels of its accumulation, analysis and dissemination, as well as the decision-making speeds concerned with it. The book “Fast Policies” by Jamie Peck and Nike Theodor was published in 2015 and the authors point out that we are witnesses of a tremendous acceleration of political dynamics. We are doing something today and the first result is needed certainly tomorrow. The idea of fast policies is to some extent the consequence of the instrumental interfaces that are used in data science for reality analysis. The change of reality seems to coincide with the speed of the necessary calculations production. You have written the code, processed your database with it, and it seems to you that reality, like this database, should be processed in seconds or at least in hours. In this sense, “fast policies” are flat in the representation of temporal modes of reality existence. The idea of a slow time disappears, but in reality everything does not always happen quickly.
The second problem, realized in Russia to some extent, is that if the data provider is a state or a corporation then the private and public balance is irreversibly disturbed. We lose the ability to distinguish between private space and public space. Theorists like Habermas or post-Habermasian theorists who exploit the democracy understanding as a publicity shared space are mistaken because the public data today doesn’t concern with the act of my conscious utterance in an institutionally regulated space, but the data on the way I regulate radiators in my apartment, or the way I set up the lighting system, or how much water I spend.
In fact, this is such a distributed and privatized publicity, condensed without my participation through technological networks. Then citizens have a choice between a retro-utopian escape from the state towards pre-technology lifestyles and the attempt to reassign centralized control and data distribution structures. The second variant is too poorly worked out, it just contains the essence of the discussion led, for example, by Eli Pariser, the author of the book “Behind the Wall of Filters” and other modern theoretical activists that discuss the possibilities to escape from large corporations power that collect almost all possible data about us and exchange it.
- What is the reason for such intense interest in Data Science? Does it concern with the possibility of collecting so much data?
Firstly, we need to understand that we are in the early stages of the data science development. In this sense, enthusiasm is really associated with the relative novelty of the possibility to collect a huge amount of data without much effort, conducting experiments not only on thousands of people, but also on millions of them that was completely impossible to do before.
The problem is that when speaking about what Big Data gives us, we cannot precisely define the word “us”. The word “we” often means the corporations that have computing resources enough to use all the features that Big Data offers. It works the same way as with no less fashionable cryptocurrencies today. Yes, you can do mining, but it requires large computational power, which is not at the disposal of some individuals. In this sense, we/Big Data is not the same as we/citizens. There are privatized "we", and the situation is that the use of Big Data is in its own substantiation. The more data we have, the more accurately we can collect still more data.
Circular logic assumes that the exclusion of something from the constant flow of Big Data carries too great risks in the context of participation in the tritest social interactions. This system was originally built asymmetrically with respect to individual users. Although it promises them a great convenience.
Big Data does not exist in a conscious way for ordinary participants of the exchange in social networks, users of search engines or consumers.
Therefore, the first thing that we need to say is to increase the level of awareness regarding the provision of your data and its analysis by a third party. The task of regaining the right to privacy is the awareness of my protection level from uncontrolled data collection about me. For example, there is the Privacy Badger application which allows you to track all the cookies that collect data on each of the websites. Big Data in the context of an individual is a challenge that needs to be realized, and something that needs to be self-determined.
- What effect does the implementation of policies based on Big Data have on society? Does the process of homogenization take place here or some kind of performative effect when reality becomes the same as the researchers saw it?
- The effect from these policies implementation is impossible to assess in the long term. The obvious thing concerning the homogenization effects is that we begin to strive for ever greater standardization within institutional procedures: to collect as many reports as possible at schools, in hospitals, corporations about all the activities of employees. This is the Big Data effect. When we talk about Big Data, we immediately have a question about the ways we will collect and generate this data.
There have already been the experiments with badges for corporate employees that analyze the quantity and quality of their communicative activity during the day. We come to a situation when the employee is in total monitoring conditions, surveillance capitalism (supervision capitalism). It is worth to afraid not only the homogenization, but also the reduction of the creativity space that is cherished by modern capitalism. If people know that each procedure is regulated, and has to coincide with a definite standard and it is within the perimeter of the information observation and collection mechanisms, they will intuitively strive to act according to the rules without violating instructions. Total legal regularity is the main effect of the oversight, supervising (although non-invasive) practices of capitalism. There is an unpleasant alternative: either we save the possibility of individual organization, but we tolerate the risk of incompetent improvisations of individual employees, and refusing the decision-making, and stress, or we imbed an extensive system for collecting information that dramatically reduces the space of an individual decision.
- Could you give an illustration of the way the problem of theory lack or context makes research false and inadequately reflecting the reality in the framework of Data Science?
- There is a good example of the assistance programs for developing countries. Many disease control programs in African countries are associated with a lack of access to basic sanitation and hygiene personal supplies. From the point of view of using Big Data there may be such a solution to this problem: you need to provide the population with sensors that show the dynamics of water quality in water basins. But as a result the population empowers these sensors with the ability to independently change the quality of this water, i.e. a tool imbedded into the data collection environment is endued with the transformation qualities of this environment. If I have such an instrument, I will start to believe that the water reservoir that I have investigated with it, and found out that it was clean, becomes pure because of this instrument. It turns out that the disease incidence associated with the water quality doesn’t become lower, but at least remains stable, i.e. sometimes it decreases, sometimes it grows. In this example, we can say that disconnect from the context is manifested in the fact that we want our tool interface always stand between the researchers and the collection objects. But the collection objects start to perceive the tool as an independent subject (the same is true for the researchers who work in the field, because the collection objects expect real changes in the situation). As a result, the communication becomes asymmetric, because the tool cannot respond, but it is addressed as if it was involved in changing reality. Perhaps this is the biggest problem and it assumes that colonialism can be specifically restored. Those ones who manage the data are deterritorialized, excluded from the perimeter where the collection objects live. The main practice of management is precise in timely deterritorialization and excluding of context. And the manager is the one who is distanced from the context.
- Are there any research examples of Data Science approach using in education?
- In the Russian context, an excellent example is the study of Ivan Smirnov, who works in the group “Data Science in Education Research” at the Institute of Education of Higher School of Economics (HSE). He collects data on VKontakte website and other social networks. His research shows that the best students get together with the best students, and the losers gather in groups with the bad students. There is the effect of homophilia here, i.e. homogeneity of the social space, which is reproduced in the digital space too. The Internet is no less a segregated space than the offline environment in contrast to the claims of its early prophets. He made an interesting study on the length of words used by the authors of the VKontakte posts in particular. It turned out that every year it grows. This is a good counter-argument for supporters of the fact that network communication causes damage to the intellectual development of young people. It is clear that the most common words in posts are the service words (like the word “really”), but a very curious fact is that the length of the used words is constantly increasing. It's another matter that Smirnov does not have the intelligible explanation. The attractiveness and the simultaneous problematic nature of data science are clearly visible, as it can generate interesting facts and predictions but it cannot inform us about the reasons why it happens.
It seems to me that there are two tasks. The first task is to make the first disconnected experiments on the study of digital traces in Russia as public as it is possible (apart from Ivan Smirnov, it is done by the group of Daniil Alexandrov in St. Petersburg), in order to give birth to the idea of what phenomena can be explored in this way. The second task is to look for the interaction ways among researchers engaged in data science with researchers engaged in more “traditional” ethnographic, anthropological areas. We should think about how we could combine the data science and the new digital ethnography. It may be worthwhile to use things that researchers are not just yet inclined to consider, such as video game cases, for example. You can be included in video games not as a player, but as a ghostly observer, who tracks everything that happens inside the game (this is a so-called machinima phenomenon: there is a character imbedded in the game, that doesn’t participate in it, but watch the action and copy the ongoing activity).
Firstly, we need to realize the scale of information that experiments with social networks allow us to collect. Secondly, we need to overcome our distrust to this, to accumulate a minimum amount of information about the ways of such data is collected and its limitations. There is a clear limitation: 75% of Facebook users have made 25 or less posts in their entire life. Most of the social networks users are silent, but the remaining 25% generate a huge amount of information, the top 5% of them is simply enormous. These differences must be understood in order to properly evaluate the information that this method of data collection gives us. Thirdly, we need to look for new strategies of the traditional development methods such as ethnography, interviews, and observation. What does “digital ethnography” mean? How to conduct surveillance online? It is interesting to raise such methodological questions today.